Closed-Loop Parcel: The Future of Shipping
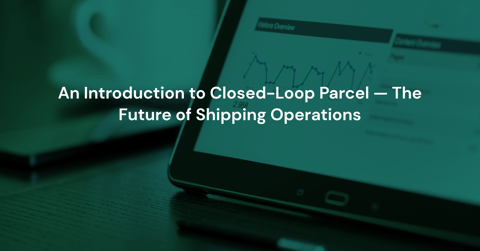
The world of parcel shipping has changed dramatically in recent years. Network complexity and customer expectations are both higher than ever.
Amazon Prime has raised the bar for performance across the board, to the point that today’s customers now expect fast, low-cost, transparent shipping options. In fact, this has become such a deeply ingrained preference that shipping is now the 2nd-greatest driver of ecommerce growth, behind only price.
Unfortunately, for many enterprise retailers, the notion of fast, flexible, low-cost shipping still presents more of a threat than an opportunity. That’s because the complexity of networks has evolved faster than their ability to keep up. There are more nodes, SKUs, carrier & service methods — as well as greater overall shipping volume.
Without the right, data-driven approach to parcel shipping, many retailers have ended up in one of two broad camps:
- Prioritizing customer experience at the cost of…costs: Retailers who prioritize offering competitive delivery promises — for example, free 2-day shipping — often end up with excess shipping costs and lower margins (not ideal, considering that shipping is already the highest operating expense on the P&L).
- Prioritizing costs and falling behind: Retailers who don’t aim to meet the increased expectation for a Prime-like shipping experience may not face the same cost pressures, but are also less equipped to deal with competitive & market pressures. Foregoing such a proven growth lever may not be the best strategy with more alternatives available to shoppers than ever before.
The retailers who have been able to redesign their parcel shipment process to meet today’s customer expectations without compromising their margins share a common characteristic — they’ve been able to effectively harness and leverage their network data to make smarter decisions at every stage in the parcel fulfillment workflow.
Data leverage is the key to meeting today’s customer expectations while improving the underlying economics of your shipping operations. While data leverage shows up in many different ways, for the purposes of Closed-Loop parcel, the most important thing is being able to use downstream data to give your operators a forward-facing view of what to expect when it comes to carrier performance & cost implications, historical transit performance and more.
In this guide, we’ll explore how to create a Closed-Loop parcel fulfillment process that enables you to make more informed decisions by leveraging the data in your network. You’ll learn about the types of data that should be (but often aren’t) used when creating delivery promises and fulfillment plans, as well as specific implications for both costs and shipping performance.
Let’s get started.
Understanding the shortcomings of current parcel fulfillment
Before exploring the concept of Closed-Loop parcel fulfillment in detail, it’s important to understand what we’re contrasting it with. While more traditional parcel fulfillment processes aren’t wholly ineffective, they present two data-related challenges that necessitate a better way of doing things.
Shippers spend more time chasing data than leveraging it
Many enterprise retailers are still struggling with fragmentation and data silos.
The use of specialized systems of record for different stages of parcel fulfillment is the right idea — but when an ecommerce platform gathers order & customer information, OMS includes inventory/product availability data, TMS includes routes & carrier performance data, and the systems don’t reliably integrate with one another, you have a problem.
Data fragmentation causes both negative process and overall business outcomes.
Process implications:
- Different departments are on different pages: The most obvious example of this is the disconnect between digital/ecommerce teams making delivery promises and the shipping ops teams responsible for executing on them. Without real data leverage, you’re likely to present a delivery promise based on static SLAs that’s either too aggressive and costly (and in some cases, not possible) to meet, or is too conservative and thus uncompetitive. This is one of the main reasons that the disconnect between these teams continues to grow.
- Supply chain visibility is limited: Operators face obscured visibility into areas for optimization and how to make sense of massive amounts of data. Rather than receiving detailed information powered by real-time integrations, they’re mostly focused on making sure that critical order information is accurately flowing downstream. This approach leaves many areas for optimization on the table, which we’ll cover in later sections.
Business implications:
- Customer dissatisfaction: Customer satisfaction can be impacted in a few different ways. On one hand, if they’re not impressed with your delivery promises for desired products (or you’re not offering delivery promises at all), you’ll see negative impacts to cart conversion and top-line revenue growth. If you make a delivery promise you don’t keep, you’re actively mismanaging expectations, which is a surefire way to negatively impact customer LTV.
- Operational inefficiencies: The lack of reliable data pipelines poses significant challenges for operational efficiency. For instance, if your digital/ecommerce teams make a delivery promise that’s too aggressive, shipping ops teams need to manage unexpected rushes and urgent deliveries that weren’t planned accurately. This leads to increased operational stress and a greater potential for errors in the fulfillment process.
- Excess costs: If a delivery promise date is too aggressive, shipping ops teams may need to expedite shipments using more expensive service methods, leave value on the table when it comes to package planning and optimization, and incur otherwise avoidable accessorial charges (ex. Dimweight charges). There will also be plenty of instances where a delivery promise may be attainable, but is met at a significantly higher cost than it needs to be. For example — in many cases, you’re likely paying for premium service methods, even though your data (if properly leveraged) could tell you that an economy method will still allow you to meet your date.
When data is siloed in loosely connected systems of record, you’re left to make decisions based on a combination of pre-defined rules, carrier SLAs, static historical performance data, and other relatively ineffective methods.
This brings us to the next overarching data challenge presented by traditional parcel fulfillment processes.
Valuable downstream data isn’t used for upstream decisions
Despite real challenges associated with excessive IT resource consumption and vendor dependency, retailers have gotten good at developing strategies and investing in technology that connects each stage of the process to the next. Data may flow from system to system without the need for too much manual correction — for instance, new order and customer data flow from an ecommerce platform to the OMS for verification and processing, then to the WMS for picking and packing, then through the TMS for carrier selection, shipment planning, and manifesting to carriers.
Here’s the problem: the latter stages of that process are generating actionable data that can be extremely valuable, but isn’t used. Some examples of this include use of actual carrier performance and cost structures, historical transit performance for different routes and seasonality etc.
This can, and often does, lead to inaccurate delivery promises, poor performance and/or excessive costs during peak, orders being routed through slower, less reliable paths, one-sided carrier contract negotiations and more.
These are the two main data-related challenges that Closed-Loop parcel fulfillment aims to solve.
Moving towards Closed-Loop parcel fulfillment
A Closed-Loop parcel fulfillment process is a system where data and feedback from various stages of the supply chain are continuously collected, integrated, and utilized to inform and optimize decisions throughout the workflow. Every stage of the process, from making a delivery promise to a package hitting a customer’s doorstop, is informed by real-time, data-driven insights — enabling you to create a competitive shipping experience that increases top-line growth without excessive costs.
In this section, we’ll explore the principles of Closed-Loop parcel, specific business outcomes you can expect by implementing it, and some of the most impactful use cases associated with it.
Principles of Closed-Loop parcel
The principles that define Closed-Loop parcel fulfillment are:
- Filling key data gaps: Systematically capturing and leveraging data from every stage in the process, from order placement to final delivery - we’ll get into specifics in the following section about use cases.
- Process automation: Ditch manual, tedious processes that burden resources. With Closed-Loop fulfillment, shippers can automate processes to drive continuous improvement in shipping execution and network optimization. No heavy lift on IT is required.
- Machine learning: As with any analytics project today, it’s not enough to simply consider what has happened in the past — you need to establish a forward-facing view of what’s likely to happen based on patterns that can be found within your data. While traditional business intelligence tools and data visualizations can help, it’s critical to build (or buy) machine learning capabilities that simplify predictive analytics and help you find “signal” within your network data.
- Continuous feedback & improvement: Rather than only leveraging downstream network data for one-off analytics projects, you can integrate it further upstream in real time to give shippers a more realistic sense for what to expect, and how they can meet customer expectations while optimizing costs.
The most important way that Closed-Loop parcel differs from traditional fulfillment is by filling key data gaps and enabling the use of downstream data in upstream decision-making and process design. Let’s explore some use cases for this in more detail.
Specific ways to better leverage downstream process data
While building a Closed-Loop system that’s designed for maximum data leverage and continuous improvement can help to fill many different process gaps, there are a few that stand out as it pertains to parcel fulfillment.
Tying delivery promises to inventory and carrier performance data
If you’re not accounting for both of these factors when making delivery promises to customers, you’re bound to either mismanage expectations or create operational and cost inefficiencies. Inventory availability is the first piece of the puzzle — after all, to make a commitment to a customer in a certain location, you need to know the closest place you have their desired SKUs in stock (whether that’s a warehouse, store, DC etc.) This gives you the understanding of origins and destinations you need to begin to measure transit performance.
However, it’s not enough to stop there. To make a truly data-backed, reliable delivery promise that doesn’t break the bank, you also need a way to predict carrier performance that goes beyond the SLAs they offer. It’s important to have an understanding of historical carrier performance against relevant criteria (ex. Types of products being shipped, locations of origins and destinations, macro-conditional data like weather patterns and fuel prices).
Choosing the most cost-effective carriers & service methods
Choosing the lowest-cost carrier at the risk of missing a delivery date is one side of the problem. But as mentioned above, if you’re relying on static SLAs to determine which carrier and service method is the best option to meet a delivery promise, you’re likely leaving significant savings on the table. In many cases, shipments that have date constraints tied to them (ex. “Delivery by Thursday”) typically don’t flow to economy service methods at all, because these more cost-effective methods don’t guarantee a delivery date.
For those reasons, one of the most impactful use cases for Closed-Loop parcel fulfillment is making intelligent downgrades based on ML-powered analysis of historical carrier performance and other relevant criteria — for example:
- Fixed network criteria like origins, operating days/times, carrier pull times, and configurable shipment options (ex. No air modes, cost-basis limits).
- Dynamic, real-time factors like time of day, day of the week, seasonality, and macro-conditions like unexpected weather events, fuel prices, or labor issues.
- Predictive factors analyzed by ML models, such as demand increases within specific periods, shipping volume trends to certain ZIP codes, and ongoing carrier performance changes/insights.
By leveraging these factors, you can effectively establish dynamic SLAs for economy service methods (ex. UPS MI), and predict delivery dates for such methods with a great deal of accuracy and confidence. Over time, this enables you to consistently downgrade to such methods and lower shipping costs while still meeting your commitments to customers.
Preparing for, and successfully executing during peak season
Peak season is the single most hectic and costly period for enterprise retailers. Demand can fluctuate wildly, leading to stockouts or overstock scenarios, ineffective route optimization and more.
With Closed-Loop parcel fulfillment, you can address that head-on.
Pre-peak, you can leverage the transit, carrier, and inventory data described above to automate and optimize carrier selection decisions as well as inventory placement. You can also proactively assess and validate peak surcharges before they begin to apply.
During peak, you can automate inventory allocation and load balancing, dynamically route orders from the optimal location, monitor carrier performance and make necessary adjustments in real-time, and scale to massive demands without completely disrupting your operations.
Closed-Loop parcel fulfillment is the future of shipping
Adopting a Closed-Loop parcel fulfillment process that’s focused on creating maximum data leverage, continuous improvement, process automation, and ML-powered predictive analytics can help your enterprise avoid the common tradeoff between meeting today’s customer expectations and running efficient, cost-effective shipping operations.
The overarching business outcomes include:
- Improved delivery accuracy and customer satisfaction: Shippers can make more accurate, data-driven delivery promises that are based on much more than available carriers & SLAs.
- Greater efficiency & more optimal shipping performance: With a greater understanding of carrier and transit performance further upstream in the parcel fulfillment workflow, shippers can make smarter decisions across inventory placement, route optimization, carrier selection & more.
- Greater cost control: By enabling smarter inventory placement, intelligent carrier & service selection, and the ability to negotiate dynamic performance-based carrier contracts rather than static volume discounts, Closed-Loop parcel helps to reduce costs throughout the lifecycle.
Put simply: by adopting a Closed-Loop parcel fulfillment process, you can improve shipping performance while lowering costs.
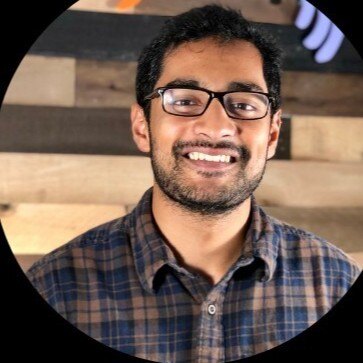
Anurag leads Shipium’s product marketing efforts, focused on helping the world understand how our platform adds incredible value for everyone.